The construction of a large-scale training dataset is essential for the development of medical image recognition systems. However, the manual labeling of images is time-consuming. Visual grounding techniques, which can detect anomalies described in the reports and automatically label them, are useful in reducing the cost of building datasets (Figure 1). Visual grounding on CT images has the following difficulties: 1) a large number of anomaly types are found on CT images, and 2) descriptions in CT radiology reports tend to be long and complex. In this paper [1], the authors proposed the first visual grounding framework for CT images that covered various body parts and anomalies. They separated the grounding task into three parts: 1) anatomical segmentation, 2) report structuring, and 3) anomaly localization described in reports (Figure 2). Anatomical Segmentation serves to extract representative anatomies (such as lung and liver) and assists the grounding model in learning detailed anatomy. Report structuring aims to identify relevant phrases for each anomaly (Figure 3). Lastly, anomaly localization outputs a localization map based on the results of the anatomical segmentation and report structuring. In the experiment, the proposed approach achieved high performance on various types of anomalies (Figure 4, 5). In the future, the use of the grounding model is expected to ease the development of medical image recognition systems.
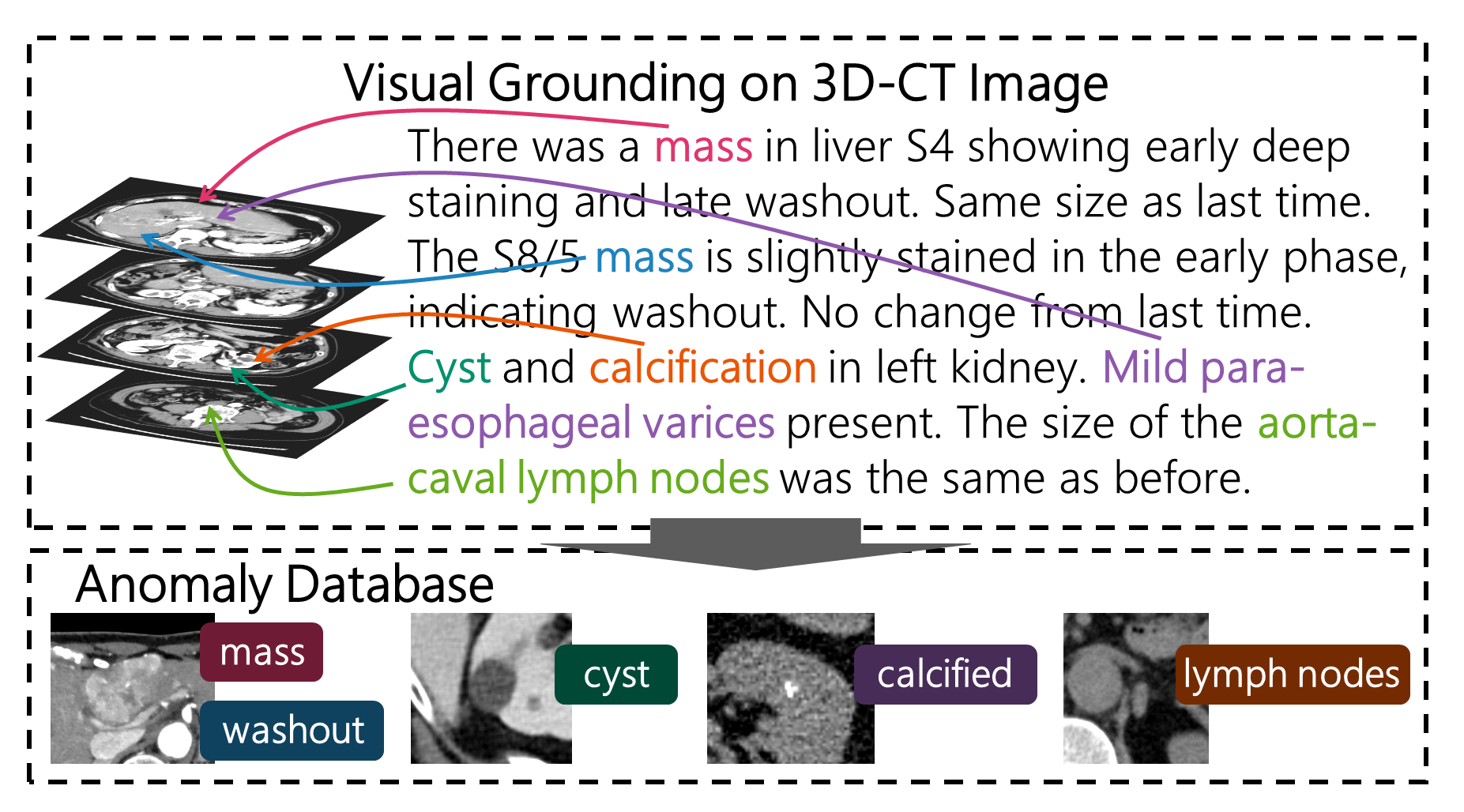
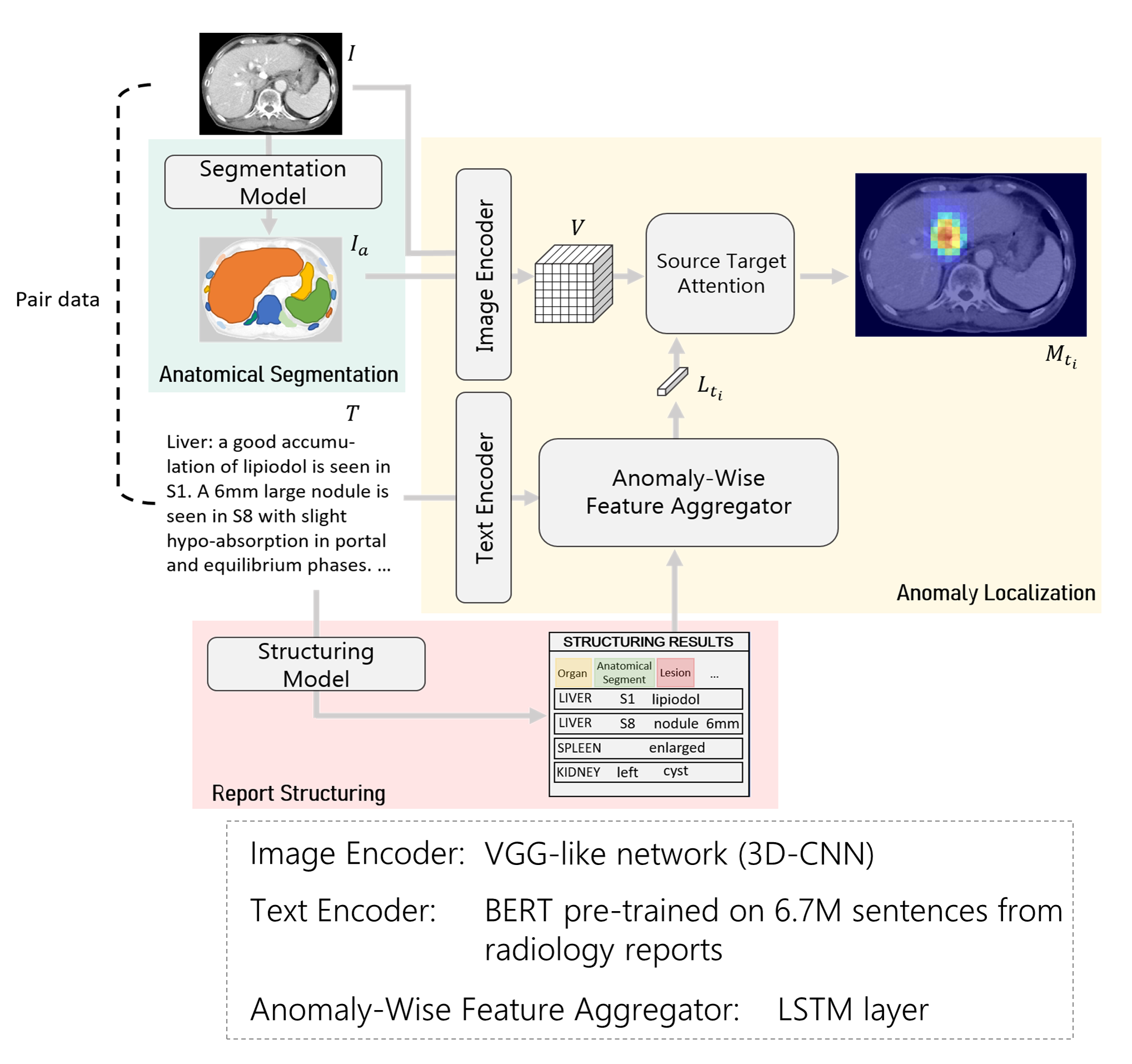
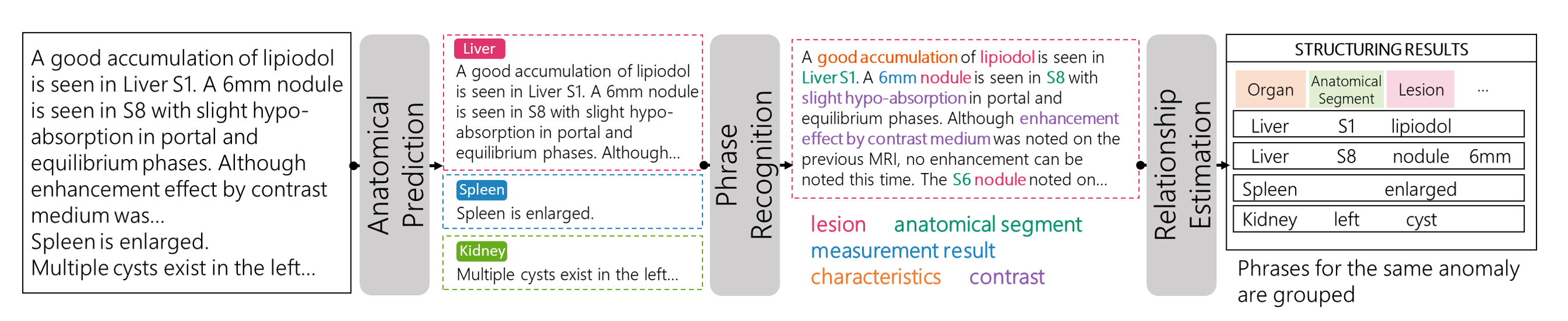
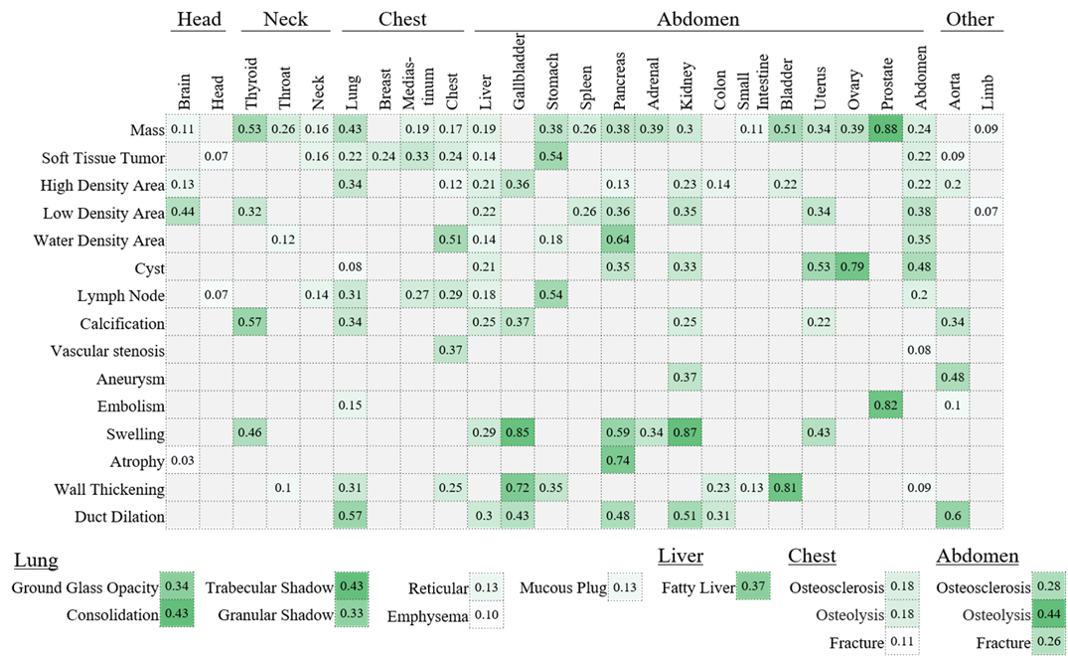
DOI: https://doi.org/10.1007/978-3-031-43904-9_59
CAUTION:This is Fujifilm Global Website. Fujifilm makes no representation that products on this website are commercially available in all countries. Approved uses of products vary by country and region. Specifications and appearance of products are subject to change without notice.