Rectal cancer is cancer that begins in the rectum that is the last several inches of the large intestine (Figure 1). Rectal cancer is one of the most common cancers and a major cause of mortality. It is important to extract cancer area and judge T-stage (Figure 2) for deciding rectal cancer treatment plans. However, it is difficult to evaluate the index from preoperative MRI images at all facilities because it requires high radiologists’ skill and experience. Therefore, this paper [1] proposed a segmentation algorithm (the mesorectum, rectum, and rectal cancer region) using deep learning so that the system can predict T-stage from segmentation results. Building such systems has two difficulties. First, preparing high quality learning datasets requires high skill and time cost. Second, advanced cancer cases are very rare. To solve these problems, the authors introduced a semi-supervised approach with a novel loss function named T-staging loss, and data augmentation of late-stage cancer using image synthesis methods (Figure 3-5). In the verification tests, the proposed method obtains the best sensitivity (0.76) and specificity (0.80) in distinguishing between over T3 stage and underT2 (Figure 6). In addition, the image synthesis-based data augmentation improved segmentation of invasion cancer area. In the future, AI will help doctors to diagnose cancer staging accurately.
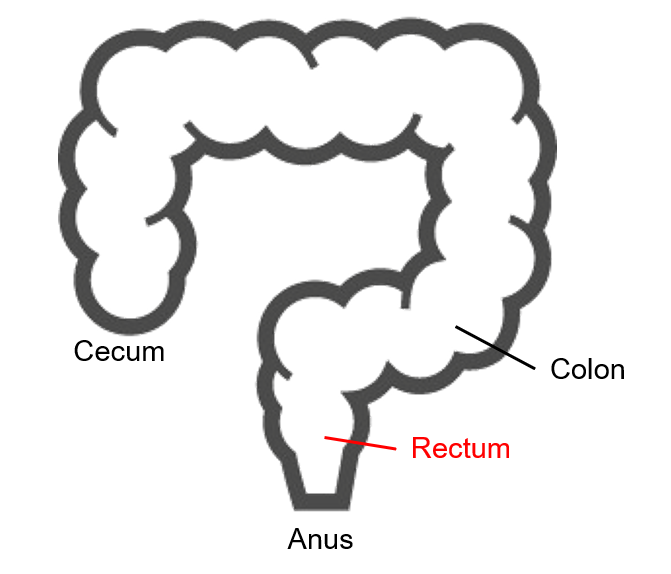
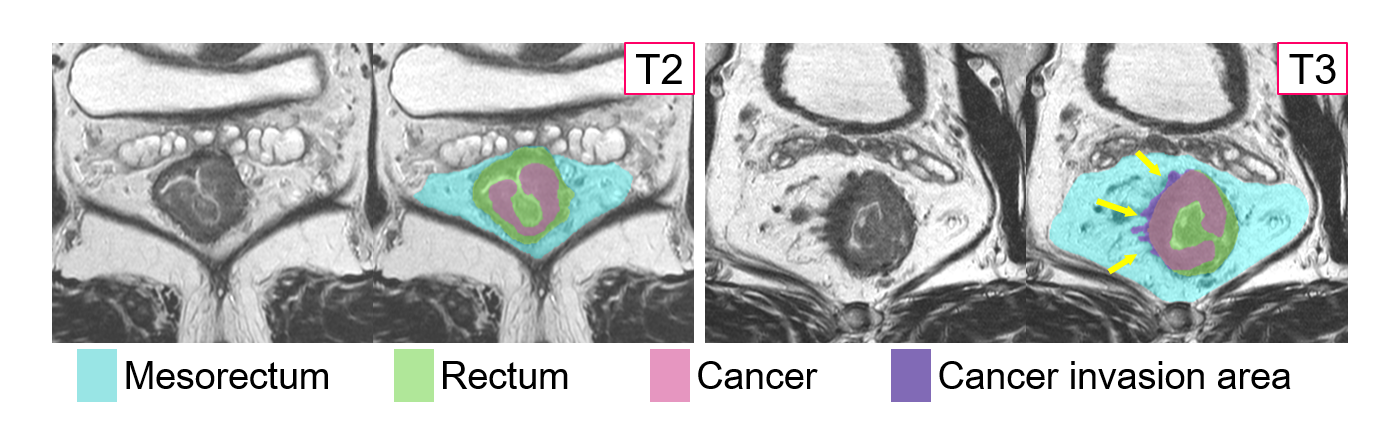
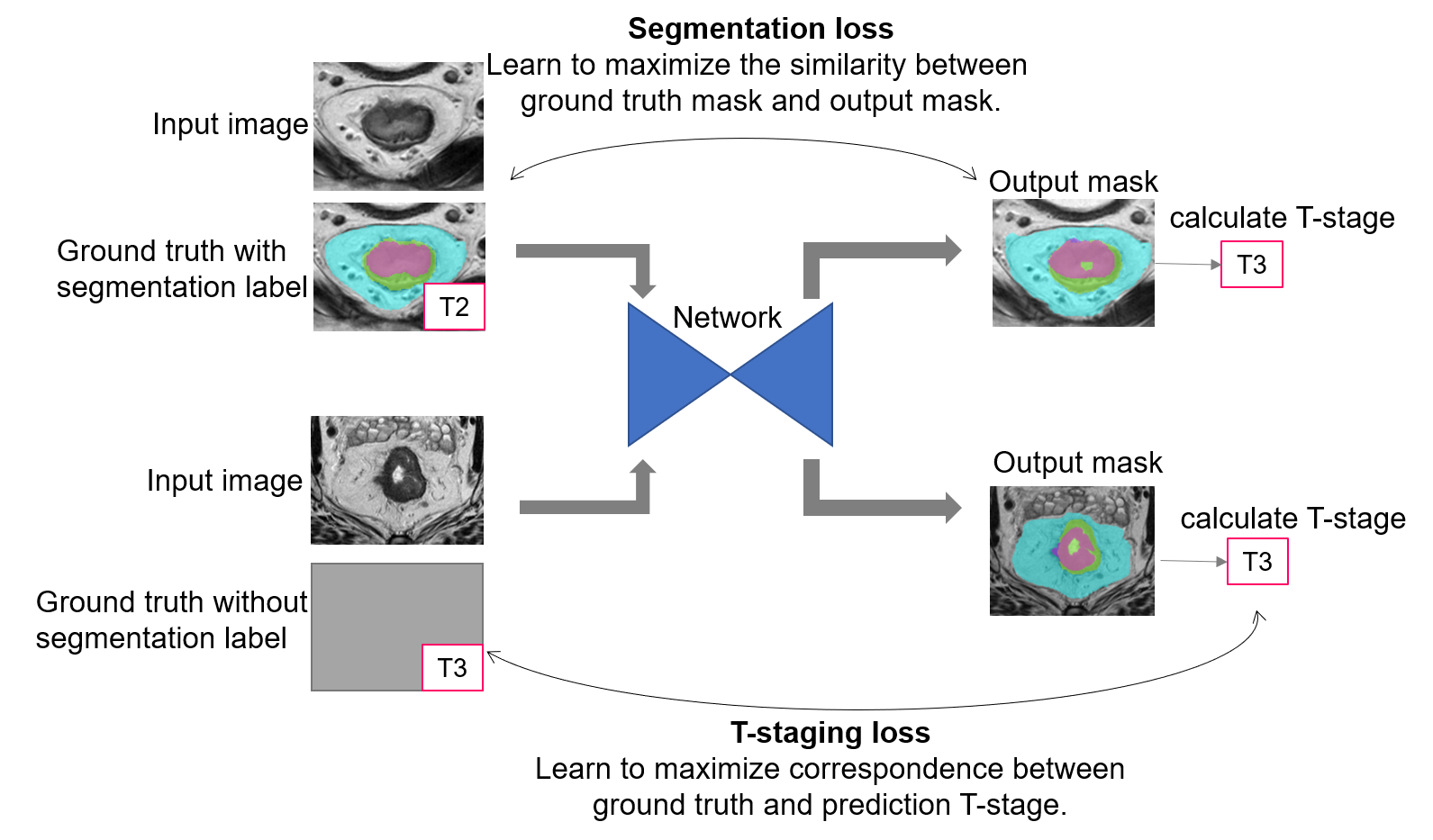
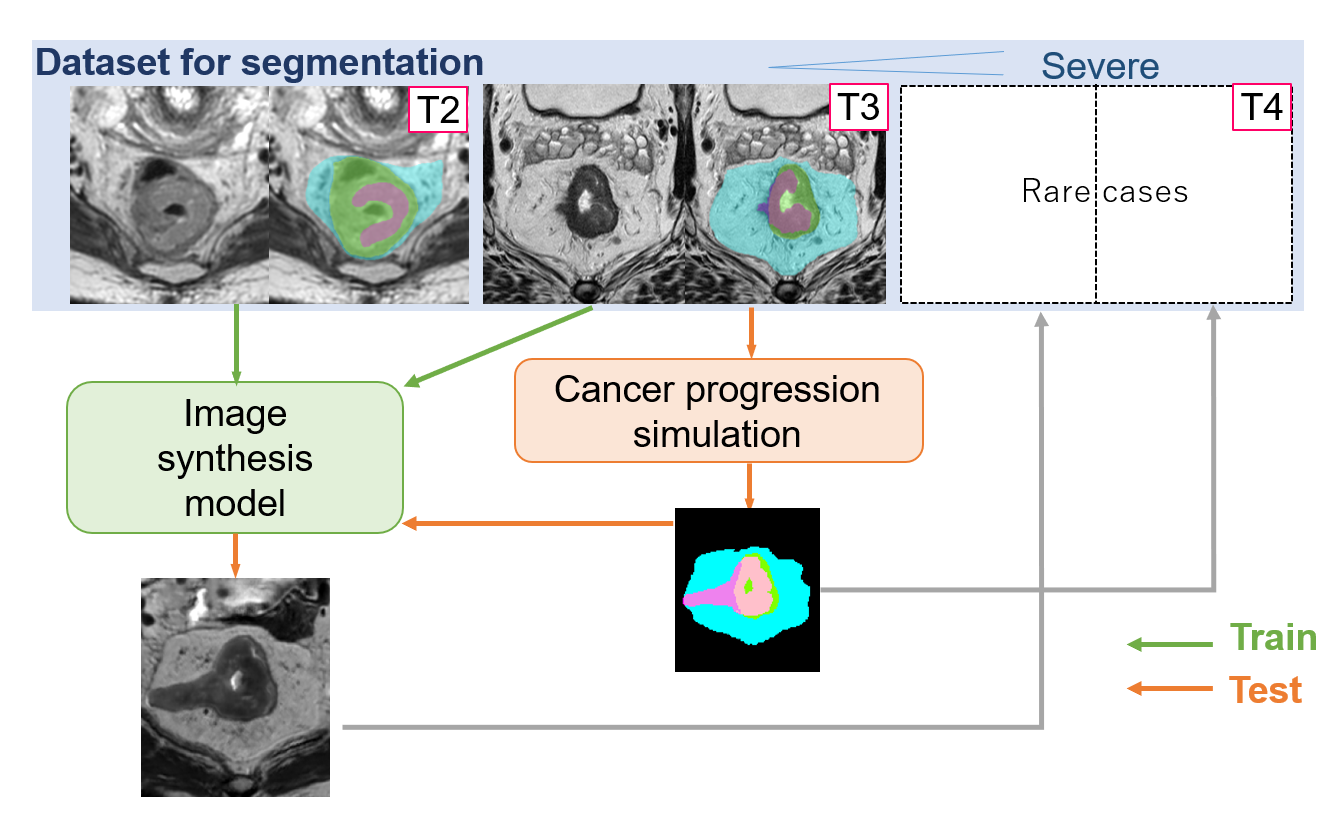
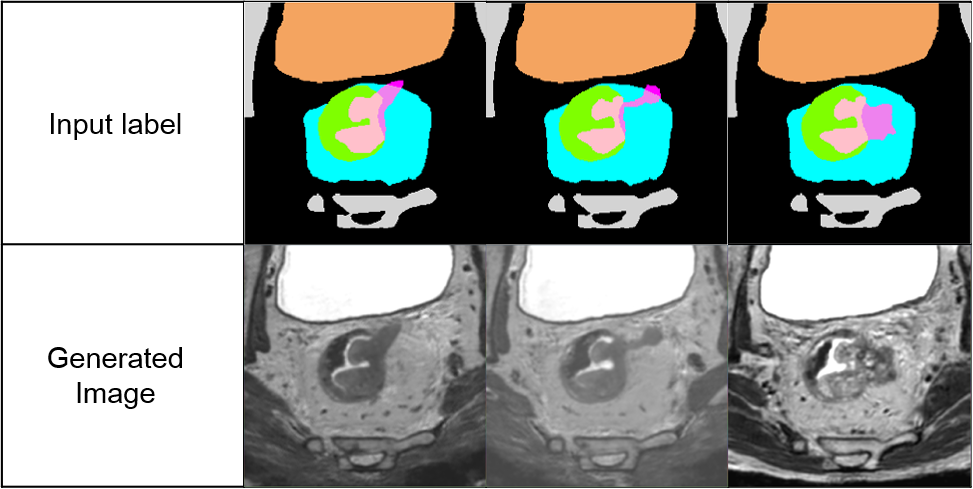
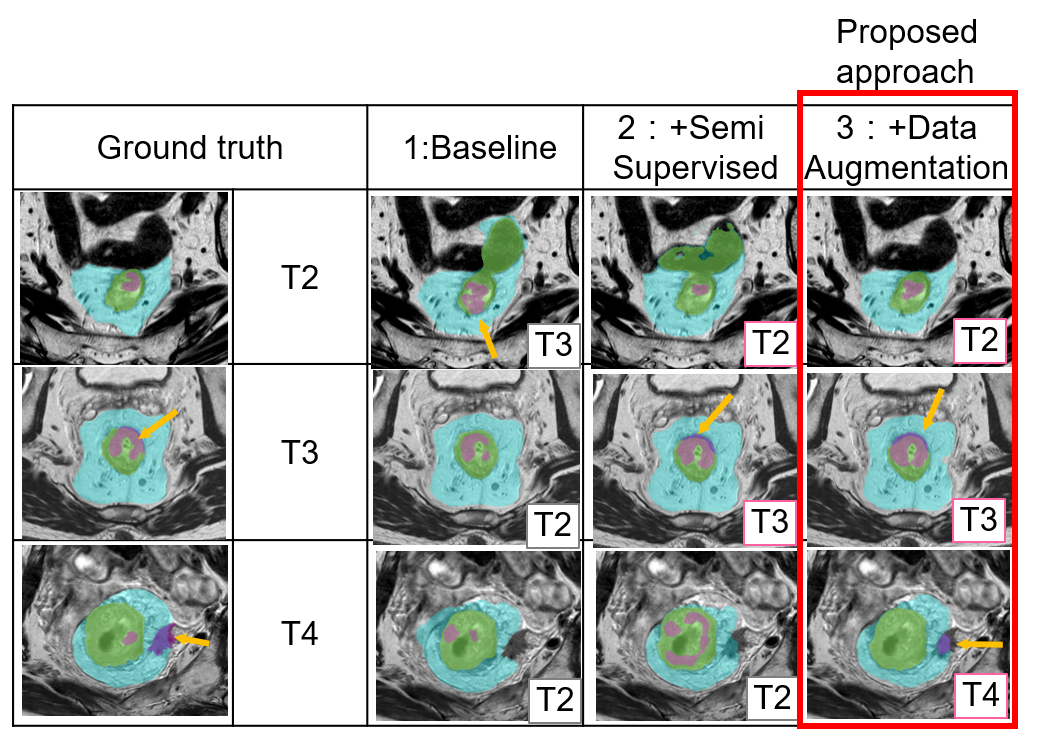
[1] Sasuga, S. et al. (2022). Image Synthesis-Based Late Stage Cancer Augmentation and Semi-supervised Segmentation for MRI Rectal Cancer Staging. In: Nguyen, H.V., Huang, S.X., Xue, Y. (eds) Data Augmentation, Labelling, and Imperfections. DALI 2022. Lecture Notes in Computer Science, vol 13567. Springer, Cham.
DOI: https://doi.org/10.1007/978-3-031-17027-0_1
CAUTION:This is Fujifilm Global Website. Fujifilm makes no representation that products on this website are commercially available in all countries. Approved uses of products vary by country and region. Specifications and appearance of products are subject to change without notice.