CT scan images can be categorized into two types: thin slice images with slice intervals are about less than 1 mm, and thick slice images with large slice intervals. Although thick slice images are frequently used for long term storage to reduce the data size, they do not have sufficient resolution for diagnosis (Figure 1). Therefore, this paper [1] proposed a method to generate thin slice images from thick slice images using a generative adversarial learning (GAN)-based algorithm. To overcome the lack of generated pattern variety, authors feed slice condition including body part information with degraded parameter scales into the discriminator (Figure 2). Experimental results showed that the proposed method obtains the best scores by objective image quality degradation tests and a subjective visible test (PSNR/SSIM metrics (Table 1) and Visual Turing Test (Figure 3)). In the future, it is expected that this method will be used as preprocessing for image recognition tasks that are difficult to process with thick slice images, and that comparative diagnosis between latest thin slice images and stored thick slice images.
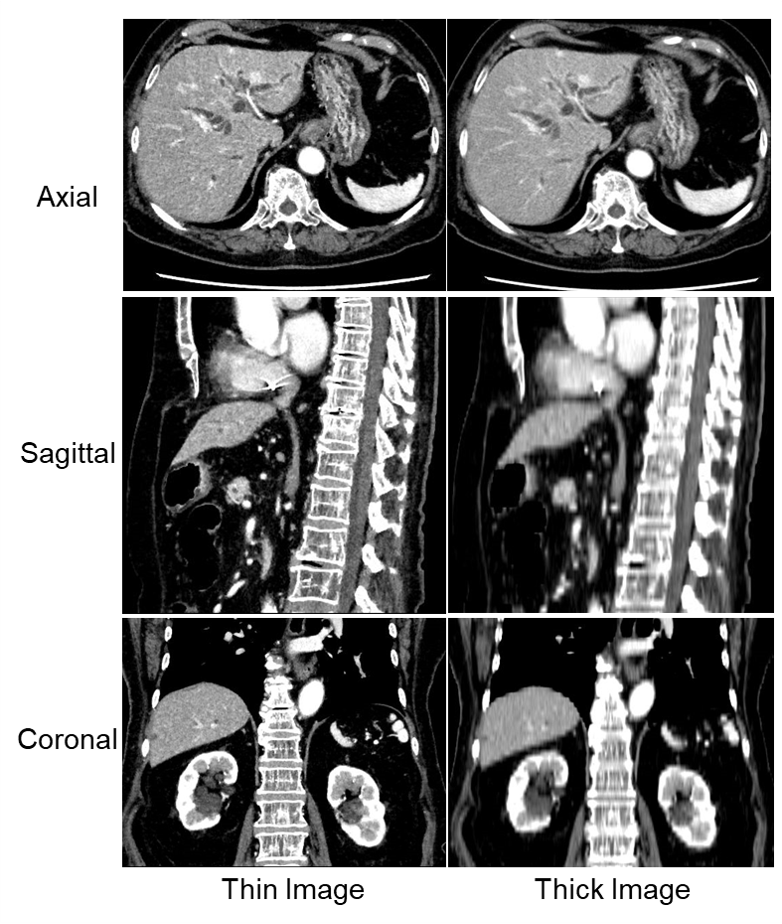
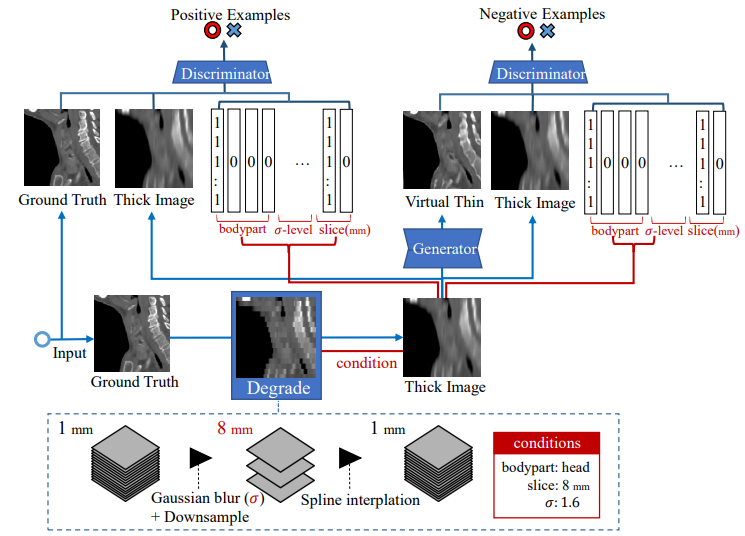
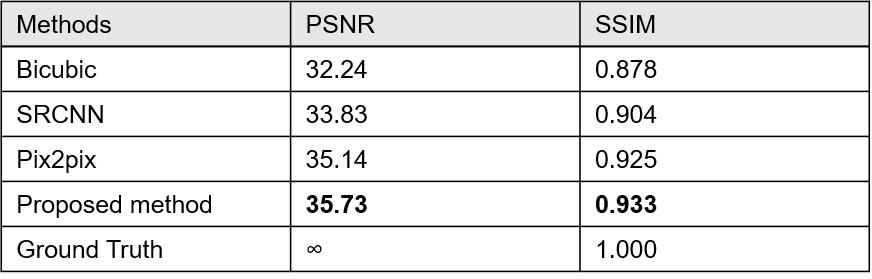
PSNR: Peak Signal to Noise Ratio
SSIM: Structual SIMilarity
(PSNR and SSIM are widely used methods to evaluate objective image quality degradation.)
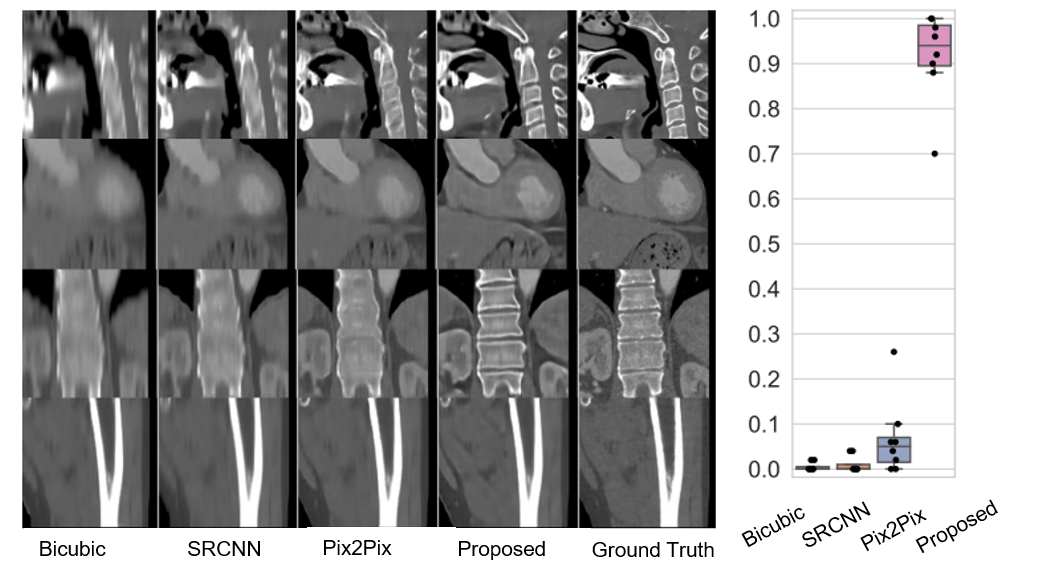
[1] Kudo, A., Kitamura, Y., Li, Y., Iizuka, S., Simo-Serra, E. (2019). Virtual Thin Slice: 3D Conditional GAN-based Super-Resolution for CT Slice Interval. In: Knoll, F., Maier, A., Rueckert, D., Ye, J. (eds) Machine Learning for Medical Image Reconstruction. MLMIR 2019. Lecture Notes in Computer Science(), vol 11905. Springer, Cham.
DOI: https://doi.org/10.1007/978-3-030-33843-5_9
CAUTION:This is Fujifilm Global Website. Fujifilm makes no representation that products on this website are commercially available in all countries. Approved uses of products vary by country and region. Specifications and appearance of products are subject to change without notice.