Radiologists use a lot of time to write medical reports manually from medical images. Automated generation of medical reports will help them to reduce their workload. However, many datasets have imbalanced distribution of finding labels (Figure 1), and it is difficult to train models accurately using those datasets. Authors introduced the clinical correctness of generated reports as a reward for reinforcement learning (Figure 2). In addition, they proposed a data augmentation to additionally train the model on infrequent findings. Experimental results showed that the reports generated by the proposed model describe the findings in the input image more correctly. Although the experiment used chest images, the method will be expected to reduce the cost of constructing learning datasets and generate reports for other body parts as well.
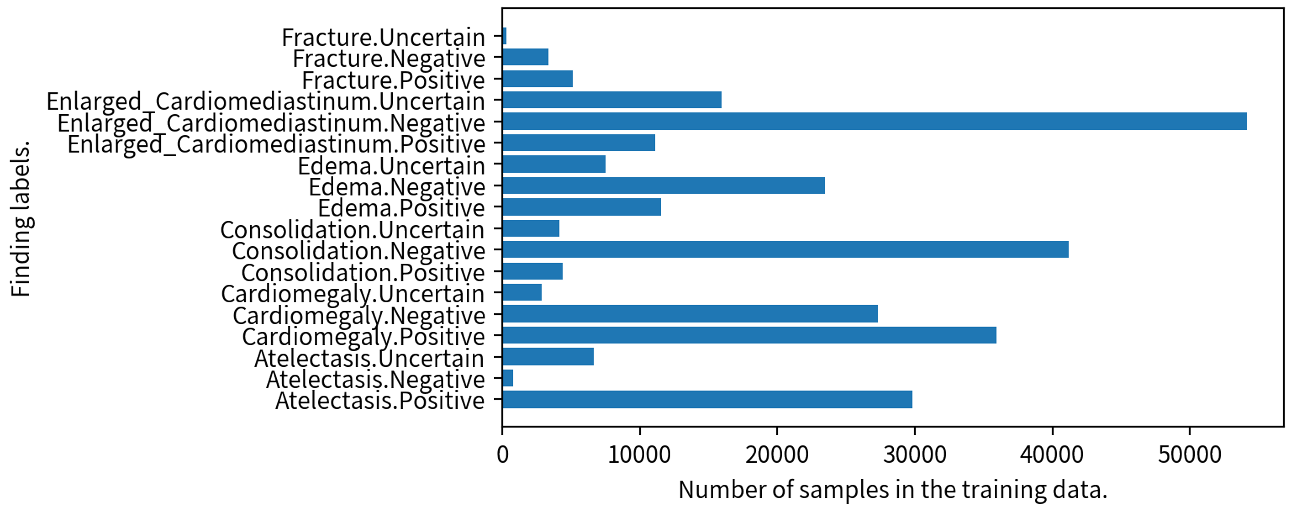
DOI: 10.18653/v1/2020.findings-emnlp.202
CAUTION:This is Fujifilm Global Website. Fujifilm makes no representation that products on this website are commercially available in all countries. Approved uses of products vary by country and region. Specifications and appearance of products are subject to change without notice.