Alzheimer’s disease (AD) which causes declination of cognitive function is the most common cause of dementia and one of the most severe social issues in the world. AD has no complete cure and treatment can only delay its progression. Therefore, it is important to detect AD in early stage and prevent it to be worse. An early stage of memory loss or other loss of cognitive ability is called Mild Cognitive Impairment (MCI) which is the stage between cognitively normal older people and AD. In this paper, the authors proposed a deep learning method to predict MCI to AD conversion using bi-linear shake fusion which computes the products of all elements between image and clinical features. The proposed method showed that higher prediction accuracy of MCI to AD conversion than the previous research. In the future, it will help screening examination of AD or deciding a stratification approach within clinical trials.
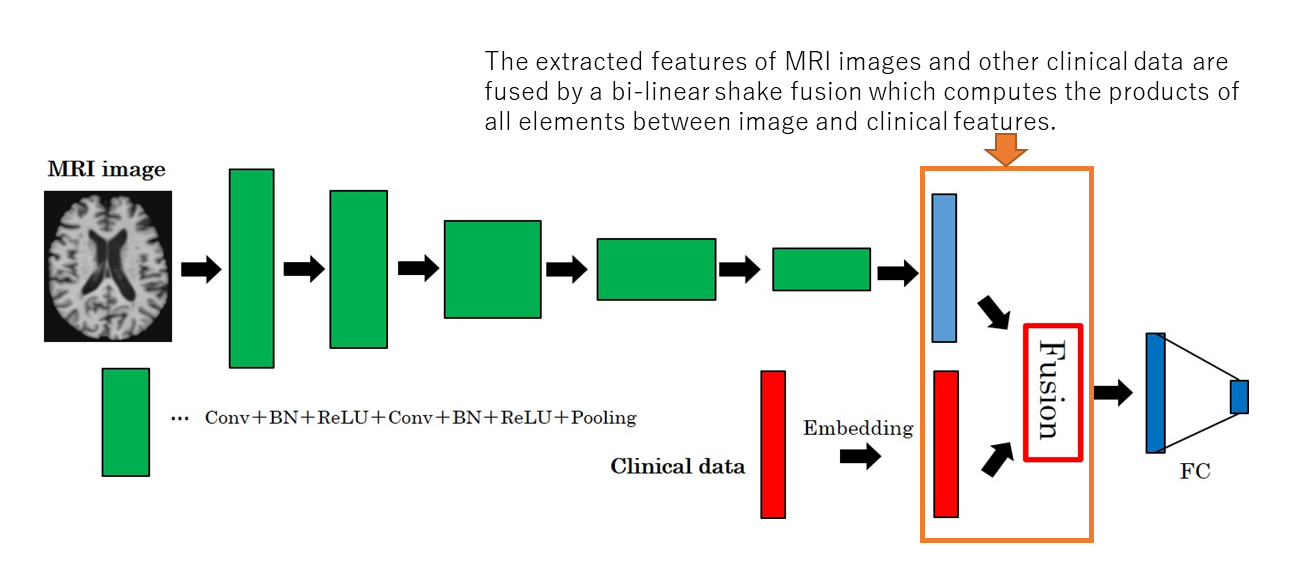
DOI: https://doi.org/10.1117/12.2549483
CAUTION:This is Fujifilm Global Website. Fujifilm makes no representation that products on this website are commercially available in all countries. Approved uses of products vary by country and region. Specifications and appearance of products are subject to change without notice.