Primary liver cancer is the third most common cause of cancer mortality. Surgery is the standard treatment method for primary liver cancer. To perform precise liver resection as a safe and curative surgery, pre-operative surgery simulation is becoming popular. A key part of preparing such simulation is reconstruction of portal and hepatic vascular structures from CT images. Although previous algorithms for automatic reconstruction of vascular structures would be to solve portal and hepatic vein reconstruction as a semantic segmentation problem using convolutional neural networks (CNN), Those have produced limited accuracy and thus require considerable manual effort in the reconstruction process because hepatic and portal vein look very alike locally in CT image crisscrossing each other (Figure 1). In this paper [1], a highly accurate hepatic and portal vein reconstruction method has been proposed. The authors proposed a learning method which learns not just to separate the vessels but puts additional constraints of topological correctness. In the proposed method, the voxels belonging to different vessels are made far in the feature space just as done in semantic segmentation. But additionally, voxels which belong to same vessel are separated by distance corresponding to geodesic distance between the voxel along the vessel centerline. This additional constraint does not allow for abrupt changes in vessel class. The proposed method achieves 7-8% improvement over previous research and can be seen that the abrupt change in classes issue is resolved with topology metric learning approach (Figure 3). In the future, it is expected that this method will make liver surgery simulation easier and lead to safe and accurate liver resection.
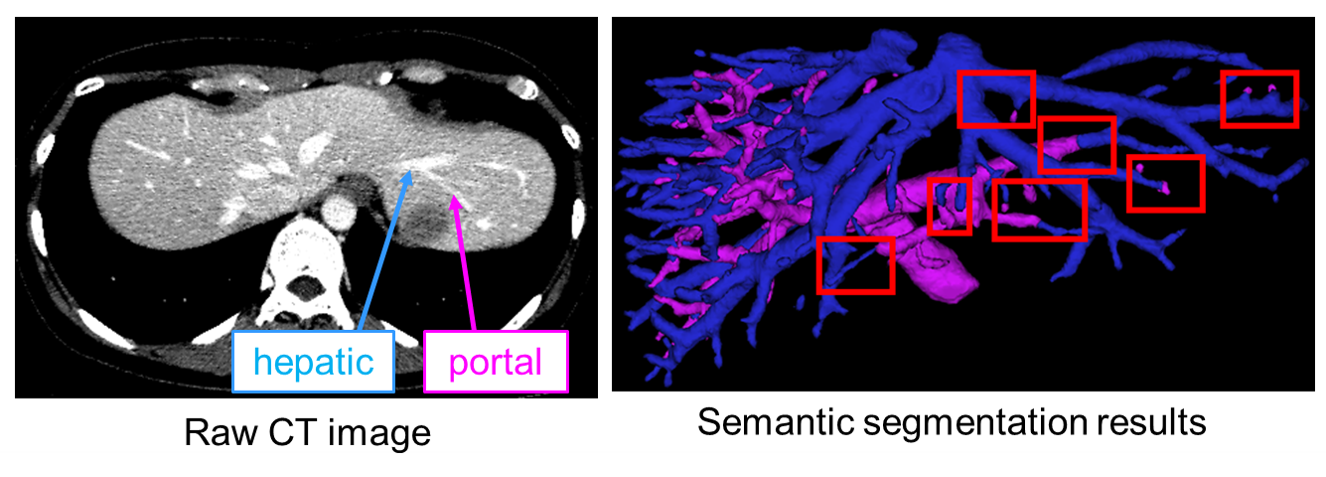
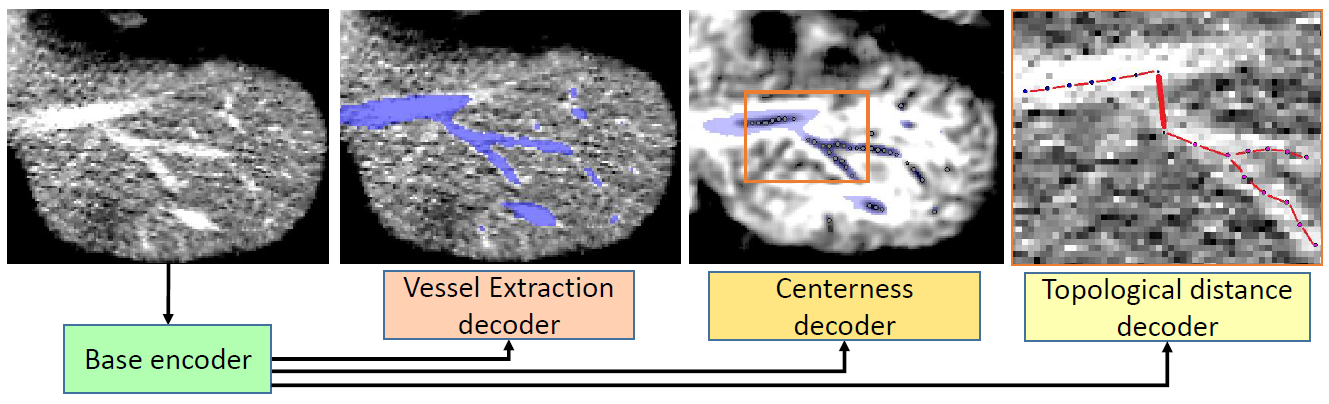
DOI: https://doi.org/10.1007/978-3-030-59725-2_2
CAUTION:This is Fujifilm Global Website. Fujifilm makes no representation that products on this website are commercially available in all countries. Approved uses of products vary by country and region. Specifications and appearance of products are subject to change without notice.