Iterative processing utilizing AI technology to enhance visibility for low dose CT images
The technology controls image quality based on a statistical model, an object model and a physical model using iterative processing.
This technology provides a high visibility image even at a low radiation dose , by maintaining the original texture of the image at a high denoise condition.
It brings both “high visibility at low dose” and “original texture at high noise reduction”
This technology provides a high visibility image even at a low radiation dose , by maintaining the original texture of the image at a high denoise condition.
It brings both “high visibility at low dose” and “original texture at high noise reduction”
Image EnhancementCTITRadiologyCardiologyRespiratoryOrthopedics
Virtual thin slice generation technology
The technology virtually generates thin slices from thick slices. It can be applied to the whole body, useful for utilizing past data. It brings high visibility in the VR display and reconstructed sagittal/coronal images.
Image EnhancementCTITRadiologyCardiologyRespiratoryGastroenterologyOrthopedics
Bone temporal subtraction
This technology visualizes the bone density temporal difference by performing image registration between the past and current image of the same patient.
The increase and decrease in CT value will be highlighted.
The increase and decrease in CT value will be highlighted.
Anatomy SegmentationCTITRadiologyOrthopedics
Pancreas analysis
Automatically extracts the pancreas, each surrounding organ area, and surrounding vessels from contrast-enhanced CT images and analyzes them in 3D.
The pancreatic duct diameter, residual pancreatic volume, measurement of the cross-sectional area, and resection simulation can be performed to support pancreatic surgery.
The pancreatic duct diameter, residual pancreatic volume, measurement of the cross-sectional area, and resection simulation can be performed to support pancreatic surgery.
Anatomy SegmentationCTITRadiologyGastroenterology
Kidney segmentation
AI technology to extract each kidney and surrounding vessels from CT or MRI images assists volume calculation of renal and compare current and past results.
This technology can also precisely extract cystic kidneys, a genetic disease that is often difficult to extract due to the diversity of shapes, and assist diagnosis and treatment.
This technology can also precisely extract cystic kidneys, a genetic disease that is often difficult to extract due to the diversity of shapes, and assist diagnosis and treatment.
Anatomy SegmentationCTMRITRadiology
Anatomic liver segments labelling
AI technology to automatically extract liver vessels and then labels liver according to the anatomic liver segments (Couinaud segments) based on the vessel running.
This can be used for Interventional Radiology planning for liver.
This can be used for Interventional Radiology planning for liver.
Anatomy SegmentationCTITRadiologyGastroenterology
Liver analysis
Technology that automatically extracts the liver parenchyma and surrounding vessels from CT images and analyzes them in 3D.
This technology can assist preoperative simulation by planning resection area based on the calculation of the dominant vessels.
This technology can assist preoperative simulation by planning resection area based on the calculation of the dominant vessels.
Anatomy SegmentationCTITRadiologyGastroenterology
Lung analysis
AI technology to automatically extract lung fields, 5 lobes, and bronchus, surrounding vessels from CT images.
From each extraction result, the percentage of low attenuation area is calculated.
These are expected to contribute the diagnosis of COPD.
From each extraction result, the percentage of low attenuation area is calculated.
These are expected to contribute the diagnosis of COPD.
Anatomy SegmentationCTITRadiologyRespiratory
Vertebrae and ribs labelling
Technology to recognize each vertebral body individually and label the vertebrae and ribs. This reduces the burden on the physician of visually counting bone positions. It is also used for visualization of fractures and bone metastasis by subtraction of rigid body alignment of vertebral bodies.
Anatomy SegmentationCTITRadiologyOrthopedics
Lung labelling
AI technology to subdivide lung into 10 segments in right lung, 8 segments in left lung from CT images. It can be used for confirming the position of chest nodules detected by a doctor.
Anatomy SegmentationCTITRadiologyRespiratory
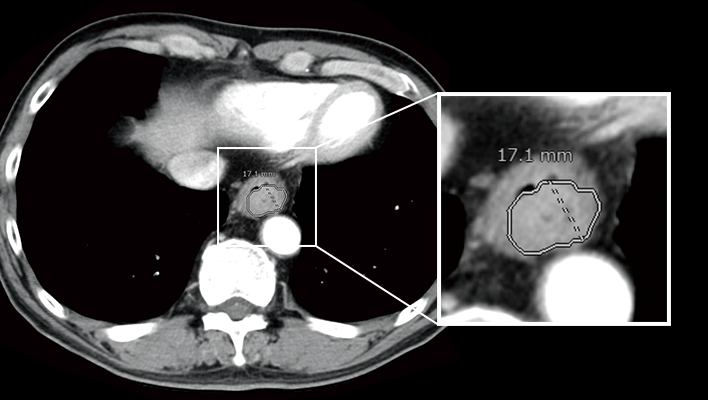
Mediastinum and axillary lymph node segmentation
AI technology to segment relatively large mediastinum, axillary lymph node from CT images. This technology can be used for assessment of lymph node enlargement.
Anatomy SegmentationCTITRadiology
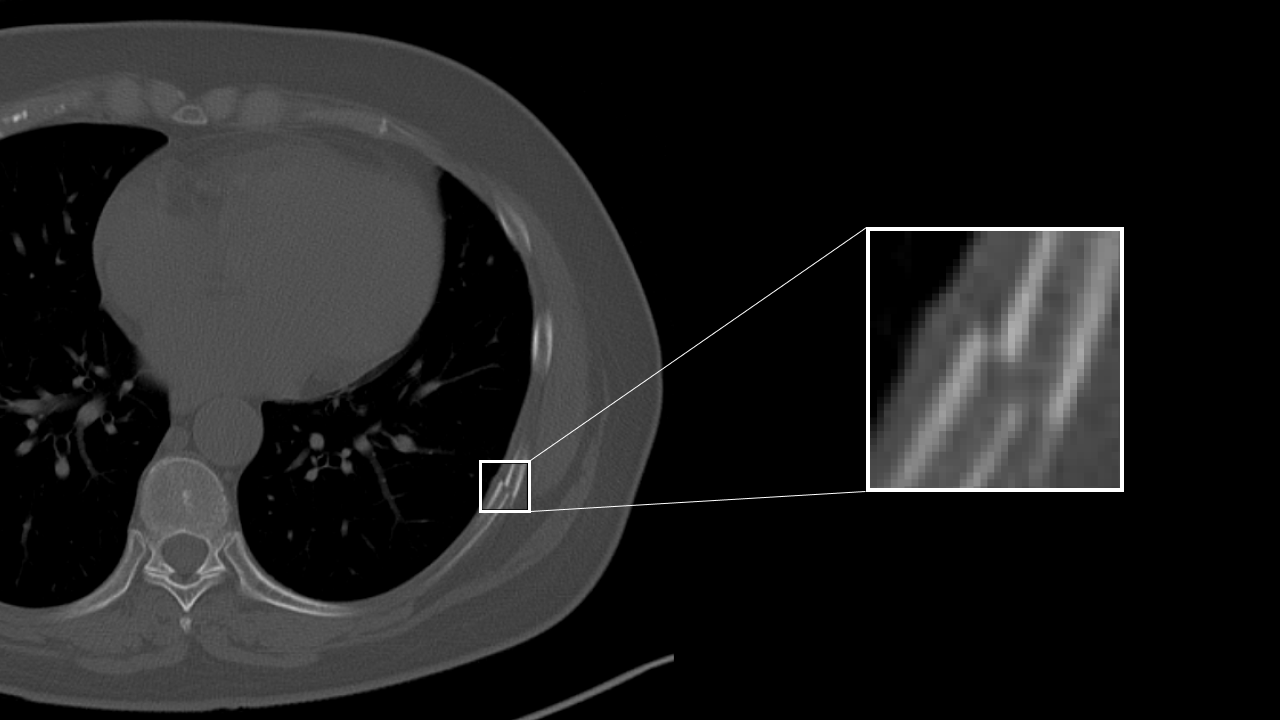
Rib fracture CAD
AI technology to detect a suspcious rib fracture from CT images. This technology will assist prevention of overlooking of subtle rib fracture.
Detection/DiagnosisCTITRadiology
COVID CAD
AI technology to identify suspicious region with COVID-19 related findings from CT images. This technology will help doctors diagnose efficiently.
Detection/DiagnosisCTITRadiologyRespiratory
Lung nodule CAD
AI technology to detect and quantify suspicious lesion from CT Images.
This will assit prevention of overlooking of nodule and generation of language of findings for radiology report.
This will assit prevention of overlooking of nodule and generation of language of findings for radiology report.
Detection/DiagnosisCTITRadiologyRespiratory
Interstitial lung disease classification
This technology identifies various findings of interstitial pneumonia that appear on CT images, such as consolidation, reticular pattern, ground glass opacity and honeycomb, and calculates their distribution and volume.
This will assist in the diagnosis of the severity and therapeutic efficacy of interstitial pneumonia, which are conventionally performed qualitatively, by providing a quantitative value for assessment.
This will assist in the diagnosis of the severity and therapeutic efficacy of interstitial pneumonia, which are conventionally performed qualitatively, by providing a quantitative value for assessment.
Detection/DiagnosisCTITRadiologyRespiratory
Highlighting areas of higher or lower signals by comparing left/right head CT images
This technology extracts high-signal and low-signal areas in head CT images by comparing the left and right sides of the brain region. Generally, high-signal and low-signal areas are used to evaluate the state of hemorrhage and ischemia in the brain for stroke diagnosis. This will assist the diagnosis of head CT imaging.
Detection/DiagnosisCTITRadiologyNeurology
Highlighting areas of higher or lower absorption than the surrounding tissue
The technology to extract and highlight areas of higher or lower absorption than the surrounding tissue. For example, high/low absorption in the liver, high absorption in the thoracic cavity, and low absorption in the kidney may be informative in determining the findings of each part. In the future, we aim to highlight both contrast-enhanced and non-contrast-enhanced images.
Detection/DiagnosisCTITRadiology
Lung nodule characterization analysis
The technology supports populating radiology report of lung nodules based on the observation and analysis results of the properties. It enables to analyze multiple properties such as shape, absorption, presence of calcification, etc., and provide multiple finding sentence candidates using natural language processing technology.
Workflow SupportCTITRadiology
Liver characterization analysis
The technology analyzes the properties of areas of interest in the liver and assists doctors in writing radiology report. It is possible to analyze not only the location and size, but also the appearance/disappearance of enhancement based on multi-phase images of contrast-enhanced CT. In addition, similar to lung nodule characterization analysis, it is also possible to present multiple finding sentence candidates using natural language processing technology.
Workflow SupportCTITRadiology
Quantification of high absorption ROI in lung field
The technology estimates high-value threshold of the region of interest, and quantify high intensity area in lung field. For example, it is expected to offer information for quantitative analysis of partially solid nodules.
Detection/DiagnosisCTITRadiologyRespiratory
Automatic measurement of EvansIndex, corpus callosum angle, and MidlineShift
This technology automatically measures EvansIndex, corpus callosum angle, and MidlineShift from head CT. EvansIndex and corpus callosum angle are expected to support the evaluation of hydrocephalus and MidlineShift to evaluate head trauma.
Detection/DiagnosisCTITRadiologyNeurology